
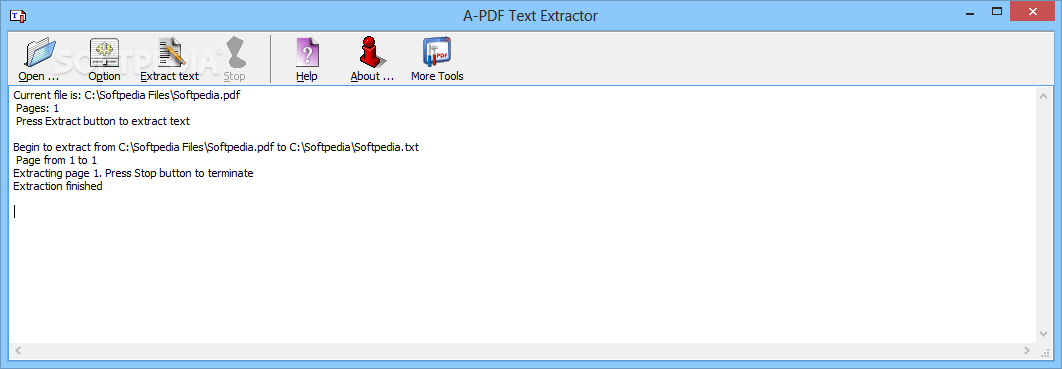
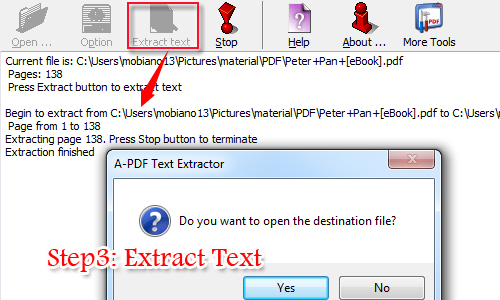
Python's PyPDF2 has an endless loop if you have a malformed PDF files with a '%' followed directly by a '\r' (as of last summer) Python's PyPDF4 handles some PDF files that pdfminer can't. Python's pdfminer handles some PDF files that PyPDF4 can't. Python's PikePDF can repair malformed and not-quite-to-spec PDF files. The libraries are pdf2image and popplerĪt work we have a lot of PDFs from a lot of different sources and authored by different systesms. Cheers!Įdit: All these are based on images, you can convert a pdf to a based on the layout, so you can use this tutorial for that. But I hope I was able to give a high-level view of what all you can do, and some possible routes. Mostly I tried out the first one as I only had a month in the company (the new semester was on my head - ). So basically you can design a pipeline where you use normal OCR for simple paragraph like data and maybe some powerful tools for table based and any other type of data.įinally, all these can be built on top of a document classifier based on the layout, so there are targeted models for a class of documents(maybe format for one company's document vs other etc). Camelot can be a good library to look into. There has been a lot of research in extracting the text from tables mostly. There are datasets like DocBank, PubLayNet etc which can be used. This is one such template to train a custom model. If you want to target the specific type of entity in a pdf, (table, figure, paragraph), you can try to extract the layout specific labels on the page, There are quite a few models based on FasterRCNN, Mask RCNN doing this. Post that, the OCR output will be much improved, any standard OCR technique from Tesseract to EasyOCR etc will give good results. Remove rows and columns from the tables, this is best done in binary page images, this is one source for starters, you can process thereafter. A simple trick is to binarize the image based on a threshold (assuming the watermarks are light enough.) For images, maybe Ghostscript can be used(there is a Python variant I couldn't find, for now, you can follow this) Remove all logos, watermarks and any other image-related stuff from the page. Trust me, the OCR performance will be really improved with a few simple image pre-processing tricks. Here is what I mostly followed:Īlways preprocess the image. Personally have worked with such a use case in my internship.
